12. Descriptive Statistics, Outliers and Axis Limits
L3 111 Descriptive Stats Outliers And Axis Limits V2
DataVis L3 11 V1
Descriptive Statistics, Outliers, and Axis Limits
As you create your plots and perform your exploration, make sure that you pay attention to what the plots tell you that go beyond just the basic descriptive statistics. Note any aspects of the data like number of modes and skew, and note the presence of outliers in the data for further investigation.
Related to the latter point, you might need to change the limits or scale of what is plotted to take a closer look at the underlying patterns in the data. This page covers the topic of axis limits; the next the topic of scales and transformations. In order to change a histogram's axis limits, you can add a Matplotlib
xlim
call to your code. The function takes a tuple of two numbers specifying the upper and lower bounds of the x-axis range. Alternatively, the
xlim
function can be called with two numeric arguments to the same result.
plt.figure(figsize = [10, 5])
# histogram on left: full data
plt.subplot(1, 2, 1)
bin_edges = np.arange(0, df['skew_var'].max()+2.5, 2.5)
plt.hist(data = df, x = 'skew_var', bins = bin_edges)
# histogram on right: focus in on bulk of data < 35
plt.subplot(1, 2, 2)
bin_edges = np.arange(0, 35+1, 1)
plt.hist(data = df, x = 'skew_var', bins = bin_edges)
plt.xlim(0, 35) # could also be called as plt.xlim((0, 35))
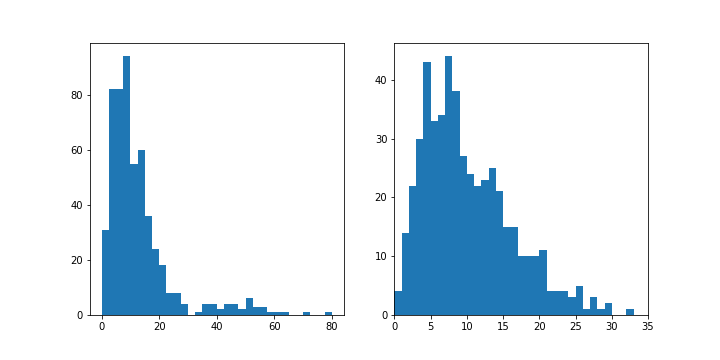
In the generic example above, we might be interested in comparing patterns in other variables between data points that take values less than 35 to those that take values greater than 35. For anything that is concentrated on the bulk of the data in the former group (< 35), use of axis limits can allow focusing on data points in that range without needing to go through creation of a new DataFrame filtering out the data points in the latter group (> 35).